Discover how data quality management (DQM) can change your financial game. With more complex and vast financial data, keeping it accurate and reliable is key. This ensures you make smart choices and follow the law. Goldman Sachs learned this the hard way, facing a $2.9 billion fine in 2020 for financial reporting issues.
Having reliable data systems is not just helpful; it’s essential. DQM boosts your financial health by cutting down on mistakes, saving time, and reducing costs. Gartner found that bad data quality costs businesses about $12.9 million a year. This shows why improving data quality is vital in finance.
Think about the benefits of making financial decisions based on solid data. Citibank, for example, uses a centralized data system for accurate customer info. As financial scenes change, using data quality management is more important than ever. Begin improving your financial data now for a brighter financial future.
Understanding Data Quality Management in Finance
In today’s fast-paced financial world, keeping data accurate is key to success. Data quality management sets standards for data quality and works to meet them. The data quality definition and the evolution of data management show how complex and vital high-quality data is in finance.
What is Data Quality Management?
Data quality management makes sure data is right, complete, and up-to-date. It involves checking and updating data regularly. It also means having strong rules and governance for data. Good data quality is crucial for smart decisions and following rules.
The Evolution of Data Quality
The evolution of data management in finance has changed a lot. In the past, managing data was simpler because there was less of it. But now, with big data and cloud storage, managing data is much harder. Financial institutions must handle data from many sources well.
The Importance of Reliable Data Systems
Having reliable financial systems is vital for success. These systems keep data accurate and on time, which helps in making smart decisions. About 66% of banks struggle with data quality, losing around $15 million a year.
Good data quality management helps avoid these losses and risks. It builds trust and keeps customers loyal. Also, 83% of banks don’t have real-time data access, showing the need for timely data solutions. By improving data checks, banks can manage risks better and follow rules more closely.
The Six Dimensions of Data Quality
Understanding the six dimensions of data quality is key to better financial data management. Making sure your data is accurate, complete, and reliable is crucial. It helps in making better decisions, reduces financial losses, and keeps you in line with rules.
Accuracy
Data accuracy means the info you use is true to life. It’s vital for smart choices and avoiding big mistakes. Companies that focus on accuracy can handle financial changes well.
Completeness
Data completeness means having all needed info in your data sets. Without it, decisions can be wrong and operations less efficient. Focusing on completeness gives a full picture of your finances, key for correct reports and analysis.
Integrity
Data integrity means your data is trustworthy and unchanged. It’s key for keeping data consistent across systems. Good integrity measures stop data problems from security breaches or bad data flows.
Validity
Data validity checks if data fits business rules and formats. Bad data can waste time and miss chances. Validity checks, like checking data types, keep data quality high.
Timeliness
Data timeliness is about how fresh and fast your data is. In quick financial worlds, timely data is essential. Focusing on timeliness boosts efficiency and quick action.
Uniqueness
Data uniqueness means each piece of data is unique, avoiding duplicates. Duplicates can mess up analysis and lead to wrong conclusions. Unique data keeps your data clear and reliable for planning and forecasting.
By focusing on these six data quality areas—accuracy, completeness, integrity, validity, timeliness, and uniqueness—financial places can improve data handling. This boosts decision-making and gives them an edge in the market.
Benefits of Financial Data Quality Management
High-quality financial data is key for today’s finance. It helps organizations make smart choices and run smoothly. This section talks about the many benefits of managing financial data well. These include better decision-making, following financial rules, and being more efficient in finance.
Enhancing Decision-Making
Having accurate and up-to-date data is crucial for making good choices. Banks and other financial places use top-notch data to understand trends and plan for the future. Bad data can lead to wrong insights and poor decisions.
When your data is good, you can trust your predictions about the market. You can also spot risks and chances for growth. This makes your decision-making stronger.
Ensuring Regulatory Compliance
Following financial rules is a big challenge for any company. Good data quality management helps keep your financial data in line with rules like Anti-Money Laundering (AML) and Counter Financing of Terrorism (CFT). Breaking these rules can cost a lot, hurt your reputation, and lead to legal trouble.
With high-quality data, companies can meet these rules better. This way, they avoid the dangers of not following the rules.
Optimizing Operational Efficiency
Good data quality can make finance work better. It cuts down on mistakes and saves time and money. Also, using automation can lower the chance of errors.
This means you can spend more time on important tasks. Good data management makes things run smoother. It helps companies use their resources wisely and avoid big problems.
Having a strong data quality management system brings many benefits. It helps with better decision-making, following rules, and being more efficient. This builds a strong base for success in the long run.
Steps to Implement Data Quality Management in Your Finance Strategy
To create a strong Data Quality Management strategy, start with a solid base. Follow these key steps to improve your data quality. This will help you get the most out of your financial data.
Conducting a Data Inventory
The first step is to do a detailed data inventory. This helps you know what data you have and how it’s used. By making a complete list of your data sources, you can find data silos and gaps that harm data quality.
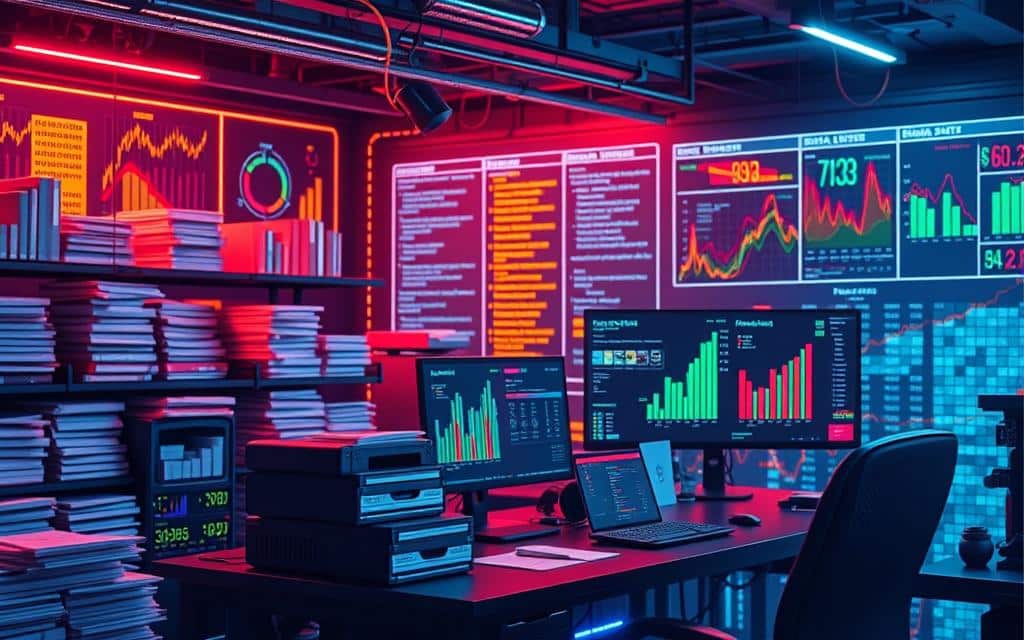
Assessing Current Performance
Then, assess how well your data systems are doing. Check if your data is accurate, complete, and reliable. These are key to finding areas that need betterment.
Use important metrics and data profiling to see your data’s health. This will show you any oddities or mistakes that need fixing.
Setting Quality Benchmarks
Lastly, set data benchmarks that match your business goals and data needs. Make clear, reachable benchmarks for data accuracy, timeliness, and consistency. Use tools for ongoing monitoring and validation to keep up these standards.
Tools and Technologies for Data Quality Management
Choosing the right tools for managing data is key in today’s fast-paced business world. These tools help keep your data accurate and reliable. This leads to better decisions and more efficiency.
Data Management Platforms
Data management platforms bring together different data sources. They make sure your data is consistent and easy to access. These platforms help process data smoothly and provide real-time analytics.
They often include tools for data profiling, cleansing, validation, and monitoring. These are essential for keeping your data accurate and consistent.
Data Governance Software
Data governance software sets rules and processes for data integrity and compliance. It fixes data issues by setting policies and standardizing data across your organization. Good data governance technologies are the foundation for strong data management and meet legal standards.
Machine Learning Monitors
Machine learning in data quality adds automation to data monitoring and upkeep. These monitors offer real-time analytics to spot and fix data problems quickly. Machine learning improves data quality across various datasets, giving you insights to keep your data systems in top shape.
Case Studies: Success Stories of Financial Data Quality Management
Looking at case studies from top financial companies shows how good data quality can change things. We’ll see how Citibank, AIG, and Visa used best practices in data management. They show how to keep data clean, reliable, and useful for making smart decisions.
Citibank’s Centralized Data System
Citibank built a strong centralized data system. It makes it easier to get data from different places. Thanks to this, Citibank’s data is more consistent and easy to understand.
This system also makes data more reliable. It helps Citibank make better choices and serve customers better.
AIG’s Data Integrity Measures
AIG has strict rules to keep its data accurate and in line with laws. They check data carefully and make sure it’s right. AIG’s focus on data integrity keeps it safe from legal problems and builds trust with customers.
Visa’s Transaction Monitoring
Visa uses smart ways to watch transactions and spot fraud. They use advanced tools to find and stop suspicious activity fast. This keeps Visa’s money safe and makes customers trust them more.
These stories show how important good data management is. They set examples for other companies to follow. They prove that clean, reliable data can really make a difference.
Common Challenges and How to Overcome Them
Financial institutions face many challenges in keeping data quality high. Knowing these challenges and how to tackle them is key. It helps protect your data and improves decision-making.
Identifying Data Silos
Data silos make it hard for departments to share information. This hurts business efficiency. To fix this, use unified data systems and encourage team talks.
Managing Data Health
Keeping data healthy is vital for financial work. Use regular checks and cleaning to manage data health. This keeps data accurate and current.
Ensuring Stakeholder Buy-In
Getting stakeholders to support data quality efforts is important. Show them how good data helps make smart decisions. Training and clear talks build a data-focused culture.
Establishing Accountability
Clear roles in data management mean everyone knows their part. Define roles and expectations. Use frameworks and check progress to keep data quality high.
By facing these challenges, financial institutions can greatly improve data quality. This leads to better analytics, more efficient operations, and smarter decisions.
How Can Data Quality Management Help in Interview Preparation for Data Management Roles?
In preparing for data management roles, mastering master data management questions for interview is crucial. Data quality management helps ensure that the data used in these interviews is accurate and reliable. By maintaining high data quality, candidates can confidently demonstrate their expertise in managing and utilizing data.
Conclusion
Mastering data quality is key for any financial institution to secure its future. By using strategic data management, institutions improve data accuracy and reliability. This leads to better decision-making, meeting regulatory needs, and boosting operational efficiency.
Data profiling, validation, and reconciliation are vital for these improvements. These steps help institutions achieve their goals.
Real-world examples show the power of good data quality management. For example, one financial organization cut data quality issues by 90% with the right strategies. This kept their financial health strong. Technology tools that automate data quality are crucial for success.
It’s important to keep monitoring and improving financial data quality. Continuous work in data governance, analytics, and automation is needed. This ensures data is collected on time and fully.
For those in finance, adopting these strategies is essential. Learn more about the right data quality strategy on this informative blog. It can help your business thrive in a competitive, data-driven world.
Securing your financial future relies on reliable data. By focusing on effective data quality management, you can use data as a strategic asset. This positions your institution for long-term success and resilience.